ChatGPT was released by the technology company OpenAI for public use on 30 November 2022. GPT-4, the large language model (LLM) underlying the most advanced version of the chatbot1, and others, such as Google’s Med-PaLM2, are poised to transform health care.
The possibilities — such as LLMs producing clinical notes, filling in forms for reimbursement and assisting physicians with making diagnoses and treatment plans — have captivated both technology companies and health-care institutions (see ‘Betting on AI for health care’).
Earlier this year, the tech giant Microsoft began discussions with Epic, a major provider of the software used for electronic health records, about how to integrate LLMs into health care. Thanks to the two companies collaborating, initiatives are already under way at the University of California San Diego Health system and at Stanford University Medical Center in California. Also this year, Google announced partnerships with the Mayo Clinic, among other health-care organizations. In July, Amazon Web Services launched HealthScribe, a generative artificial intelligence (AI) clinical documentation service. And venture-capitalist firms have invested US$50 million in a US start-up called Hippocratic AI, which is developing an LLM for health care.
In the rush to deploy off-the-shelf proprietary LLMs, however, health-care institutions and other organizations risk ceding the control of medicine to opaque corporate interests. Medical care could rapidly become dependent on LLMs that are difficult to evaluate, and that can be modified or even taken offline without notice should the service be deemed no longer profitable — all of which could undermine the care, privacy and safety of patients.
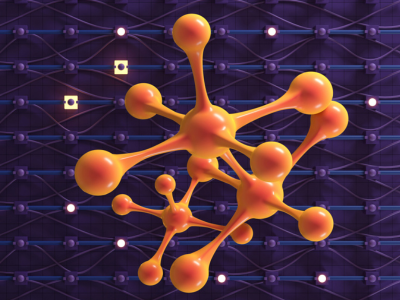
Science and the new age of AI: a Nature special
Although technology companies dominate in terms of resources and processing power, health-care systems hold a powerful asset — vast repositories of clinical data. Also, thousands of hospitals and institutions worldwide are now investing millions of dollars in disparate efforts to integrate AI into medical care. In an executive order on AI that US President Joe Biden signed last month, several organizations, including the US Department of Health and Human Services and the US Department of Veterans Affairs, have been tasked with investigating how to safely implement AI in health care3. In the United Kingdom, the National Health Service has allocated more than £123 million ($153 million) to the development and evaluation of AI, and a further £21 million to its deployment. Similarly, in June, the European Union allocated €60 million ($65 million) to research for AI in health care and its deployment.
By pooling their resources and expertise, such organizations could develop LLMs that can be transparently evaluated and that meet local institutional needs — even if they are also working with corporations. Specifically, these organizations could develop open-source models and software tailored for health care, and then fine tune these base models to create privacy-compliant, locally refined models that incorporate privately held data. In other words, carefully governed open collaboration between diverse stakeholders could steer the development and adoption of LLMs so that AI enhances medicine rather than undermines it.
The promise and pitfalls
Typically, the first step in training an LLM involves feeding the model massive text-based data sets from the Internet, to produce a base model. This initial training period requires considerable engineering expertise and vast computing power. The pre-trained model is then trained further on higher-quality curated data sets, and specialists assess the model’s output to ensure that it is accurate and aligns with relevant safety protocols and ethical norms. This expert feedback can even be used to train the model further. For example, ChatGPT has been fine-tuned to give users the experience of having a human-like conversation.
Some LLMs have shown impressive capabilities in the medical domain2,4,5. In March last year, Microsoft researchers described how GPT-4, which has no medical-specific training, can pass certain medical tests, including the United States Medical Licensing Examination5. In July, two of us (A.T. and B.W.) co-authored a study in which we found that clinicians often preferred clinical notes that were generated by GPT-4 to those generated by physicians6. Other work has shown that GPT-4 can pass examinations in some specialist areas, such as neurosurgery7 and medical physics8. Studies have also demonstrated the impressive abilities of LLMs in diagnosing challenging cases9,10 and in translating complex surgical consent forms into language that can be easily understood by patients11.
Yet, despite the promise of LLMs to improve the efficiency of clinical practice, enhance patients’ experiences and predict medical outcomes, there are significant challenges around deploying them in health-care settings.
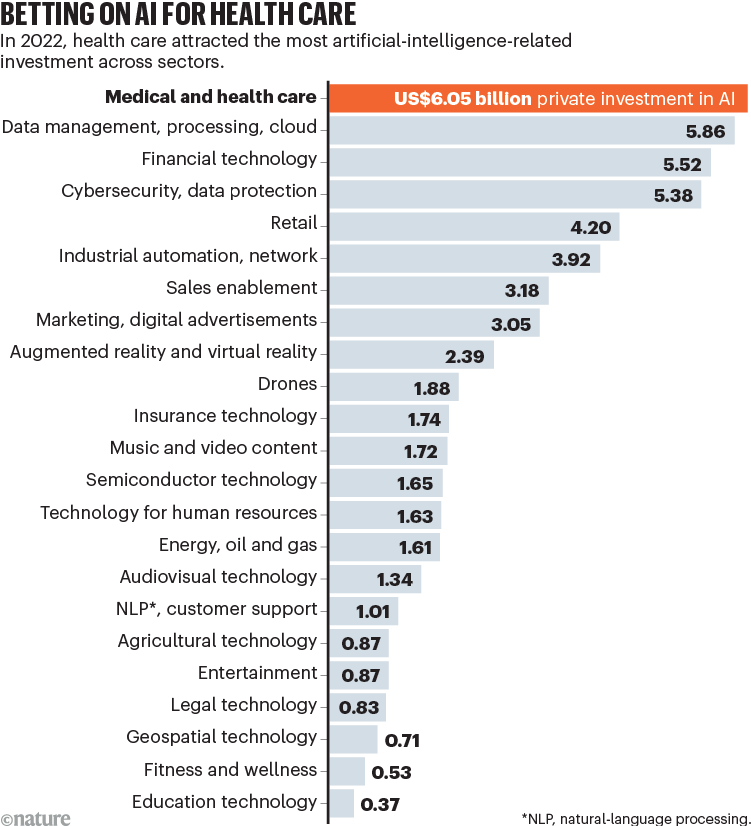
LLMs often generate hallucinations — convincing outputs that are false12. If circumstances change — for example, because a new virus emerges — it is not yet clear how a model’s knowledge base (a product of its training data) can be upgraded without expensive retraining. If people’s medical records are used to train the model, it is possible that with the relevant prompts, the model could recreate and leak sensitive information13 — particularly if it is trained on data from people with a rare combination of medical conditions or characteristics.
Because the models are products of the vast swathes of data from the Internet that they are trained on, LLMs could exacerbate biases around gender, race, disability and socioeconomic status14. Finally, even when those studying LLMs have access to the base models and know what training data were used, it is still not clear how best to evaluate the safety and accuracy of LLMs. Their performance on question-answering tasks, for example, provides only a superficial measure that doesn’t necessarily correlate with their usefulness in the real world15.
Safe integration
As long as LLMs are developed in relative secrecy, it is especially difficult to envision how this technology could be safely integrated into health care.
Many LLM providers, including OpenAI, use a closed application programming interface (API). This means the instruction from the user (to produce a clinical note from a transcribed conversation between a patient and a physician, for example) and the data from the user (the transcribed conversation) are sent to an external server. The model’s outputs are then returned to the user. With this approach, users often do not know the exact model or method that is processing their request. Typically, the user does not know what data the model was trained on or whether the model was modified between their uses of it16. In some cases, it is unclear what happens to the data provided by the user and how those data are protected from being accessed or misused by others.
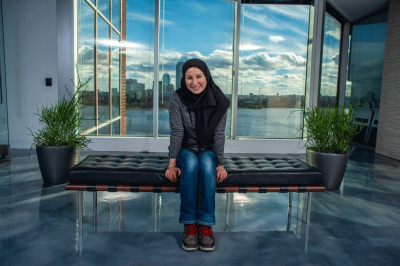
ChatGPT one year on: who is using it, how and why?
Partly in response to complaints from users, OpenAI stated in March that it would make any one version of its LLMs available for three months so that users can have consistent access to the same models for at least this period. What other providers are doing concerning model updates is unclear. Moreover, many models might have been trained on the questions that are then being used to evaluate them. Yet, because the developers of many proprietary models do not share the data sets their models are trained on, the degree to which this kind of ‘contamination’ is occurring is unknown.
Another problem specific to proprietary LLMs is that companies’ dependency on profits creates an inherent conflict of interest that could inject instability into the provision of medical care. This was demonstrated recently by the UK health-tech company Babylon Health, which promised to combine “an artificial-intelligence-powered platform with best-in-class, virtual clinical operations” for patients.
When it went public in 2021, Babylon Health was valued at more than $4 billion. After complaints about its services and other problems, and reportedly costing the UK National Health Service more than £26 million in 2019, the company filed for bankruptcy protection for two of its US subsidiaries in August this year.
All in all, it is hard to see how LLMs that are developed and controlled behind closed corporate doors could be broadly adopted in health care without undermining the accountability and transparency of both medical research and medical care.
Open models
What’s needed is a more transparent and inclusive approach.
Health-care institutions, academic researchers, clinicians, patients and even technology companies worldwide must collaborate to build open-source LLMs for health care — models in which the underlying code and base models are easily accessible.
What we’re proposing is similar to the Trillion Parameter Consortium (TPC) announced earlier this month — a global consortium of scientists from federal laboratories, research institutes, academia and industry to advance AI models for scientific discovery (see go.nature.com/3strnsu). In health care, such a consortium could pool computational and financial resources as well as expertise and health-care data.
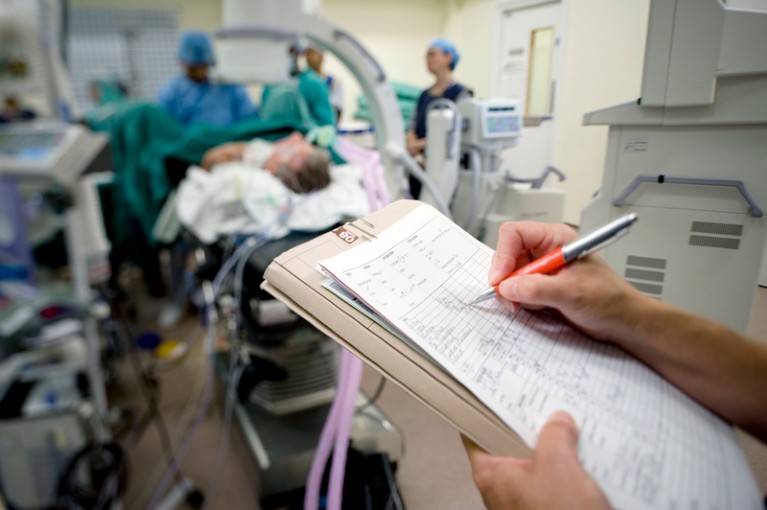
This consortium could build an open-source base model using publicly available data. Consortium members could then share insights and best practices when fine-tuning the model on patient-level data that might be privately held in a particular institution. Alternatively, to save the considerable costs associated with the first phase of training LLMs, consortium members could work together to improve open models that have already been built by corporations.
It is encouraging that some organizations have committed to making their LLMs more accessible. For example, for both LLaMA (Large Language Model Meta AI)17, which was publicly released by technology company Meta in February (although its status of ‘open-source’ is debated by some), and Mistral 7B18, an LLM released by the French start-up Mistral AI in September, users can download the models and fine-tune them using their own data sets. This means that users can probe the performance of the models on a deeper level than is currently possible with closed LLMs such as GPT-4.
Some people might question whether a global consortium would have enough resources to build LLMs from scratch. The computing time needed to build GPT-3, a precursor to GPT-4, is estimated to have cost around $4.6 million. But the potential cost savings from AI in the US health-care sector alone is projected to be between $200 billion and $360 billion annually. Also, thanks to advances in hardware and techniques, the cost of training high-quality models is rapidly falling.
And with their access to vast troves of clinical data, health-care institutions, governments and other consortium members have a significant advantage over technology companies. This, combined with it being easier to use such data for non-commercial uses, means that consortium members are well positioned when it comes to curating high-quality clinical data that could be used to improve LLMs.
Such an open consortium-led approach provides several advantages over the development of proprietary LLMs for medicine. First, testing LLMs across multiple consortium organizations would help to ensure their reliability and robustness. In principle, clinicians, machine-learning specialists and patients could collectively and transparently contribute to the evaluation of models — similar to how volunteers contribute to editing entries of the free online encyclopedia Wikipedia or how researchers contribute to the review of scientific papers.
A future ideal would be for consortium members to share any patient-specific data that they use to fine-tune LLMs, should they find ways to do so safely. In the meantime, with local institutional control over data, it will be easier to ensure that patient-privacy and other requirements are met. By coordinating efforts, LLMs can be integrated into electronic health-record systems, such as health-care company Oracle Cerner’s platform, Epic and other systems that are already widely used by hospitals and health-care institutions. Also, designers and engineers can optimize models as well as ways to evaluate them and user interfaces without reinventing the wheel each time.
Up for debate
All sorts of issues need thrashing out. To protect patient privacy, stringent guidelines for how clinical data can be used and measures to prevent data leaks will be crucial. LLMs must be adjusted to reflect variations in institutional requirements and varying health-care practices and regulations across different countries and regions. Steps will need to be taken to guard against LLMs being used to exacerbate inequity, and to mitigate harm from inappropriate use of LLMs, such as for self-diagnosis and treatment.
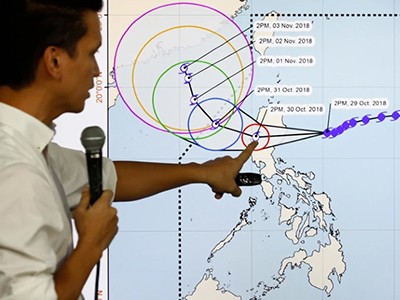
AI will transform science — now researchers must tame it
At least in relation to data sharing, various efforts offer some guidance. The MIMIC (Medical Information Mart for Intensive Care) database contains unidentifiable information for people admitted to a medical centre in Boston, Massachusetts. External researchers can use the data if they complete a training course in human-subjects research and sign a data-use agreement. Other successful platforms for sharing health data include the UK Biobank, a biomedical database containing genetic and health information from half a million UK participants. In some cases, federated learning, a method in which groups enhance a shared AI model using their data without exchanging it, could be instrumental19.
But for many of these challenges, a range of strategies will need to be considered. In fact, it is precisely because the use of LLMs in medicine poses such formidable challenges around safety, privacy and equity that those at the front line of care should drive the development and deployment of the models. Whereas transparent efforts could provide a solid foundation for AI in medicine, building medicine on the top of proprietary, secretive models is akin to building on a house of cards.
"control" - Google News
November 30, 2023 at 11:06PM
https://ift.tt/2BDJ53h
Generative AI could revolutionize health care — but not if control is ceded to big tech - Nature.com
"control" - Google News
https://ift.tt/Oh3UK5H
https://ift.tt/jqtXwN1
Bagikan Berita Ini
0 Response to "Generative AI could revolutionize health care — but not if control is ceded to big tech - Nature.com"
Post a Comment